
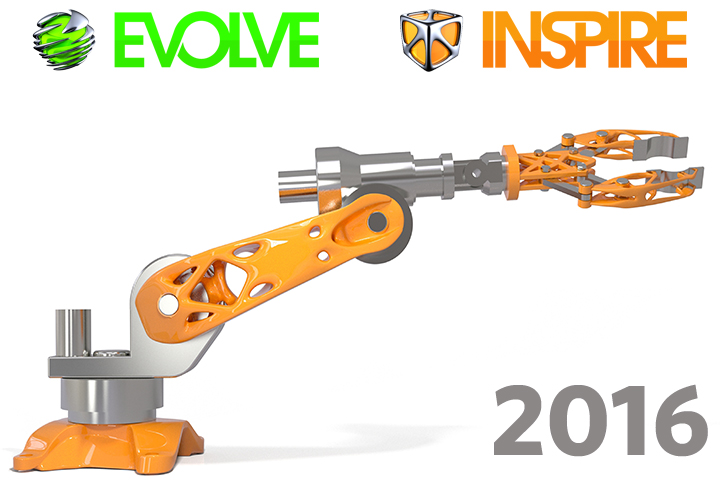
Concretely, we use a cyclical approach involving multiple stages (1) training an ensemble model of multiple U-Net architectures with the provided high confidence hand-labeled data and, generated pseudo labels or low confidence labels on the entire unlabeled test dataset, and then, (2) filter out quality generated labels and, (3) combine the generated labels with the previously available high confidence hand-labeled dataset. We propose a semi-supervised learning pseudo-labeling scheme that derives confidence estimates from U-Net ensembles, thereby progressively improving accuracy. The NASA Impact Emerging Techniques in Computational Intelligence (ETCI) competition on Flood Detection tasked participants with predicting flooded pixels after training with synthetic aperture radar (SAR) images in a supervised setting. Journal of Inverse and III-posed Problems de Gruyter Flood Segmentation on Sentinel-1 SAR Imagery with Semi-Supervised LearningĪbstract: Floods wreak havoc throughout the world, causing billions of dollars in damages, and uprooting communities, ecosystems and economies. A sensitivity analysis completes the study and evinces the method's higher susceptibility to perturbations in the surface topography than in surface mass-balance or rate factor. On real-world inspired geometries, it is shown to produce estimations of even better quality in smaller time than the recently proposed steady and transient inverse methods.

Novelty of the presented shape optimization algorithm is the use of surface topography and mass-balance only within a time-dependent Lagrangian approach for moving-boundary glaciers. Neither filtering of the surface topography where its gradient vanishes nor interpolation of the basal shear stress is involved. The approach is based on the minimization of the surface topography misfit at the end of the glacier's evolution in the shallow ice approximation of ice flow.

Michel, Laurent Picasso, Marco Farinotti, Daniel Bauder, Andreas Funk, Martin Blatter, HeinzĪbstract We present a shape optimization algorithm to estimate the ice thickness distribution within a two-dimensional, non-sliding mountain glacier, given a transient surface geometry and a mass-balance distribution. Estimating the ice thickness of mountain glaciers with a shape optimization algorithm using surface topography and mass-balance Estimating the ice thickness of mountain glaciers with a shape optimization algorithm using.
